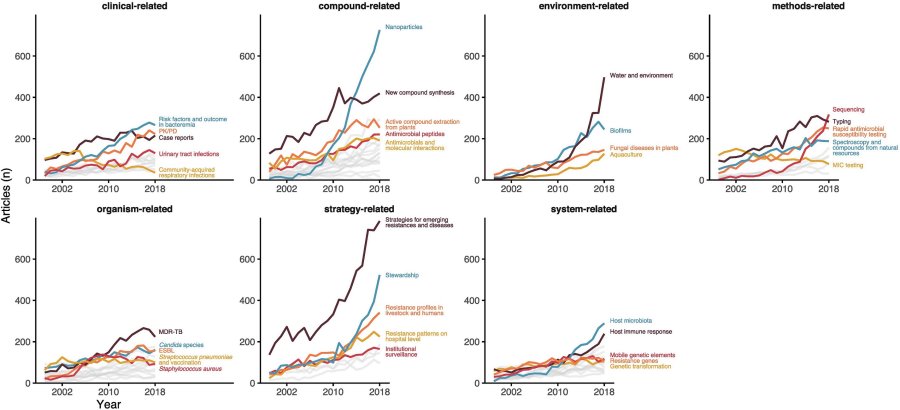
Antimicrobial research is a vast, constantly evolving field, making it difficult to keep track of trends and new topics. In a recent study, researchers from the Netherlands attempted to overcome this problem by using machine learning to classify more than 150,000 AMR research articles into automatically-generated topics. For today’s Spotlight, let’s take quick look at how the AMR world has changed over the last two decades.
In this study, Luz et al used structural topic modelling, which analyses articles and automatically suggest topics to group them into. They automatically processed more than 150,000 AMR research articles between 1999 and 2018 into 88 topics – think of it like a massive, but very simplified, systematic review. The annual evolution of the top 10 topics in AMR research by total papers published is shown in GIF form in our newsletter this week. The data is also broken down in the header picture of this Spotlight, showing the new articles per topic per year, sorted into thematic groups (clinical, compound…).
Let’s discuss a few insights we can get by staring at these images. Firstly, we see that “Strategies for emerging resistances and diseases” consistently remains the dominant topic. This is a clear sign that AMR is definitely not an old problem, but rather an evolving one, requiring constant rethinking of how to best tackle it. To echo this, “New compound synthesis” is always in second place of the ranking by total articles published (GIF), reflecting ongoing efforts to address the discovery void in new antibiotic classes since 1987.
The other spots in the top 10 list are much more fluctuating. For example, the importance of antibiotic stewardship has increasingly gained recognition in recent years, as can be seen by the exponential increase in new “Stewardship” articles since 2010 (picture). Similarly, there is a clear recent spike in “Nanoparticles” articles, a sign of the potential of this field to provide new solutions against AMR. Based on the trends in numbers of new articles per year (picture) on “Water and environment”, “Sequencing”, and “Host microbiota”, we can also assume that these topics will claim spots in the top 10 list in upcoming years.
Finally, it’s important to note that although “MDR-TB” (multidrug-resistant tuberculosis) entered the top 10 list in 2012 (GIF), the number of new articles per year on that topic has been decreasing since 2016 (picture). This is worrying, as progress in tuberculosis mortality has been reversed by the COVID-19 pandemic, and the proportion of MDR-TB cases is likely increasing.
Although these observed trends in AMR research are insightful, they come with the usual caveats of machine learning (some arbitrary choices required, risk of misclassification…). The authors have tried to minimise this by checking each automatically proposed topic manually, adjusting them if necessary, and discussing with up to five reviewers. Overall, this work is a promising starting point to identify gaps in AMR research and inform future work. The complete dataset if openly available here if you want to take a look!
Article discussed: Luz CF, van Niekerk JM, Keizer J, Beerlage-de Jong N, Braakman-Jansen LA, Stein A, Sinha B, van Gemert-Pijnen JE, Glasner C. Mapping twenty years of antimicrobial resistance research trends. Artificial intelligence in medicine. 2022 Jan 1;123:102216. https://doi.org/10.1016/j.artmed.2021.102216
LSHTM's short courses provide opportunities to study specialised topics across a broad range of public and global health fields. From AMR to vaccines, travel medicine to clinical trials, and modelling to malaria, refresh your skills and join one of our short courses today.